BETTER CLOUD PERFORMANCE USING CACHED TRANSACTIONS AND DTNS
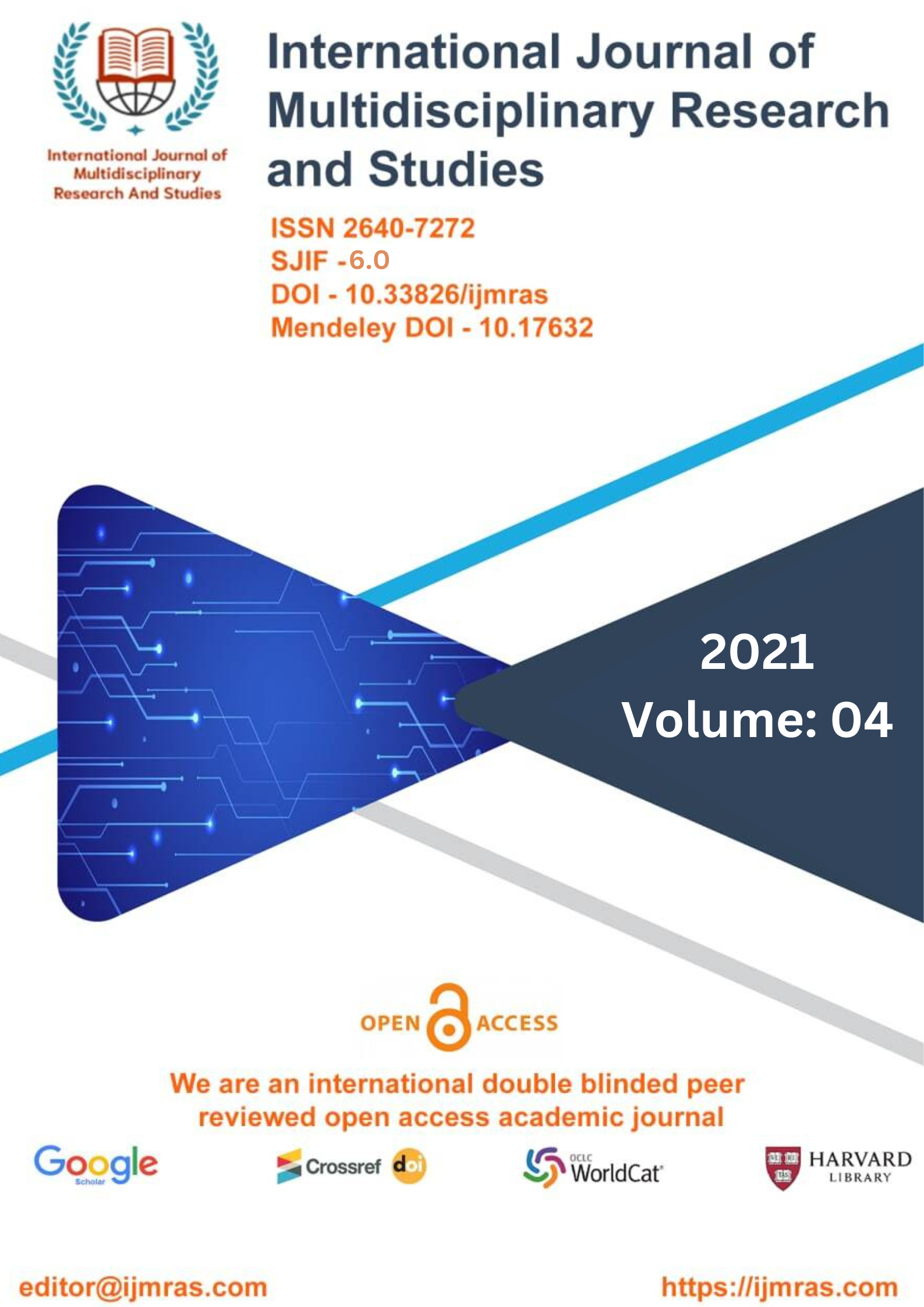
Abstract
The term "mobile cloud computing" refers to a new computing paradigm that was developed to increase the capabilities of mobile devices. This kind of computing has been gaining more and more attention in recent years. Existing research focuses mostly on how to make optimal use of the computing power of an individual device by using the processing power of faraway cloud data centers or the capabilities of a local mobile cloud established by devices in the immediate vicinity. In contrast to the studies that have been done before, our research focuses on the question of how to enhance the performance of data sharing in a peer-to-peer mobile cloud, despite the existence of a restricted bandwidth as well as a dynamic and unpredictable wireless channel state. To be more specific, we first formulate the data transmission between devices as a utility maximization problem with the consideration of limited bandwidth, incentive participation, and the QoE (Quality of Experience) heterogeneity, based on incorporating a publish/subscribe component into the base station. This formulation takes into account the fact that there is a limited amount of bandwidth available. After that, a dynamic online method is built that does not need the future context (for example, channel status) of the mobile cloud. This algorithm is used to make the choice of data transmission and the selection of the communication interface concurrently. An exhaustive theoretical study demonstrates that the suggested algorithm is both optimum and successful in achieving its goals. Extensive testing is carried out in order to validate the findings of the study and demonstrate that the suggested algorithm is superior to the techniques that are already in use.
Keywords
DTNS, performance, cloud, computing, algorithm, heterogeneityHow to Cite
References
M. Armbrust et al., “Above the clouds: A Berkeley view of cloud computing,” EECS Department, University of California, Berkeley, Tech. Rep. UCB/EECS2009-28, Feb. 2009.
S. Das, D. Agrawal, and A. El Abbadi, Elastras: an elastic transactional data store in the cloud, in USENIX HotCloud, 2009.
A. J. Lee and M. Winslett, “Safety and consistency in policy-based authorization systems”, in ACM CCS, 2006.
M. K. Iskander, D. W. Wilkinson, A. J. Lee, and P. K. Chrysanthis, “Enforcing policy and data consistency of cloud transactions,” in IEEE ICDCS-SPCC, 2011.
Islam, M.A., Vrbsky, S.V., Tree-Based Consistency Approach for Cloud Databases," in IEEE Second International Conference on Cloud Computing Technology and Science (CloudCom), 2010.
United States Road Assessment Program. http://www.usrap.org. Accessed: 2018-1-13. 12
traffic safety facts Technical Report, National Highway Traffic Safety Administration, 2016. 1
Global status report on road safety 2018. Technical Report, Geneva: World Health Organization, 2018. License: CC BYNC-SA 3.0 IGO. 1
Rashalshehi and Prashanth Reddy Marpu. Hierarchical graph-based segmentation for extracting road networks from high-resolution satellite images. ISPRS Journal of Photogrammetry and Remote Sensing, 126:245–260, 2017.
Sean M Arrieta, Alexi A Efros, Ravi Ramamurthy, and Manish Agarwal. City forensics: Using visual elements to predict non-visual city attributes. IEEE Transactions on Visualization and Computer Graphics, 20(12):2624–2633, 2014. 7
Phavenbastani, Songtao He, SophianAbbar, Mohammad Alizadeh, Hari Balakrishnan, Sanjay Chawla, Sam Madden, and David DeWitt. RoadTracer: Automatic extraction of road networks from aerial images. In Proceedings of the IEEE Conference on Computer Vision and Pattern Recognition, pages 4720–4728, 2018. 23
Francois Cholet. Exception: Deep learning with deeply separable resolutions. Correspondent, ABS/1610.02357, 2016 25
Marius Cordes, Mohamed Omran, Sebastian Ramos, TimoRehfeld, Markus Enzweiler, Rodrigo Bennsen, Uwe Franke, Stefan Roth and Bernd Schiele. Cityscapes dataset for semantic urban scene understanding. In IEEE Conference on Computer Vision and Pattern Recognition, 2016. 6
Abhimanyu Dubey, Nikhil Naik, Devi Parikh, Ramesh Raskar and Cesar A. Hidalgo. ´ Deep Learning the City: Quantifying Urban Perception on a Global Scale. In European Conference on Computer Vision, 2016. 7
Andreas Geiger, Philipp Lenz and Raquel Urtasun. Are We Ready for Autonomous Driving? Kitty Vision Benchmark Suite. In IEEE Conference on Computer Vision and Pattern Recognition, 2012. 7
Connor Greenwell, Scott Workman, and Nathan Jacobs. Where it goes: Predicting object distribution from above. 23 in IEEE International Geoscience and Remote Sensing Symposium (IGARSS), 2018
Douglas Harwood, Karin Bauer, David Gilmore, Reginald Soularete, and Zachary Swan. Validation of the US road assessment program star rating protocol: application to the safety management of US roads. Transportation Research Record: Journal of the Transportation Research Board, 2147:33–41, 2010. 7, 12, 23
License
Copyright (c) 2021 AMRENDRA KUMAR

This work is licensed under a Creative Commons Attribution 4.0 International License.
Individual articles are published Open Access under the Creative Commons Licence: CC-BY 4.0.